In the ever-evolving world of technology, Artificial Intelligence (AI) is making its mark in every domain, and mathematics is no exception. With its ability to process vast amounts of data and complex algorithms, AI has the potential to revolutionize the way we understand and interact with numbers. In this article, we will explore the promising impacts of AI in the realm of mathematics and how it is reshaping the future of this age-old discipline.
AI-powered algorithms are capable of solving complex mathematical problems at lightning speed, enabling mathematicians to tackle challenges that were once considered impossible. Beyond solving equations, AI is also being used to develop new mathematical theories, uncover patterns, and make predictions in various fields, including finance, engineering, and physics.
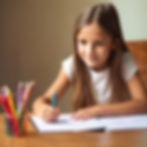
Moreover, Artificial Intelligence in Maths is enhancing the teaching and learning experience by providing personalized education, adaptive learning platforms, and intelligent tutoring systems. Students can now access personalized feedback, recommendations, and resources tailored to their individual needs, accelerating their understanding and retention of mathematical concepts.
Join us as we delve into the world of AI and its promising impacts on mathematics, uncovering the exciting possibilities and pathways it offers for the future of numbers.
The role of AI in solving complex mathematical problems
AI-powered algorithms are capable of solving complex mathematical problems at lightning speed, enabling mathematicians to tackle challenges that were once considered impossible. Traditionally, mathematicians have relied on manual calculations and intuition to solve intricate equations and mathematical puzzles. However, with AI, these complex problems can be solved more efficiently and accurately.
One area where AI has shown remarkable progress is in the field of optimization. Optimization problems, which involve finding the best solution from a set of possible options, are notoriously difficult and time-consuming to solve. AI algorithms, such as genetic algorithms and simulated annealing, can explore vast solution spaces and quickly converge on optimal or near-optimal solutions. This capability has practical applications in various domains, including logistics, resource allocation, and scheduling.
Moreover, AI has also proven to be invaluable in solving mathematical problems that require extensive computation or analysis. For example, the Four Color Theorem, which states that any map can be colored with at most four colors in such a way that no two adjacent regions have the same color, was famously proved with the help of AI. The proof involved analyzing billions of possible configurations, a task that would have been impossible without the computational power of AI.
In summary, AI's ability to solve complex mathematical problems quickly and accurately is revolutionizing the field of mathematics, enabling mathematicians to explore new frontiers and push the boundaries of what is possible.
AI-powered mathematical modeling and simulations
Mathematical modeling and simulations play a crucial role in various scientific and engineering disciplines. They help researchers understand complex systems, make predictions, and test theories. With the advent of AI, these modeling and simulation processes have become more sophisticated and powerful.
AI algorithms can analyze vast amounts of data and extract meaningful patterns and relationships. This capability is particularly useful in developing mathematical models that accurately represent real-world phenomena. By feeding data into AI algorithms, researchers can uncover hidden patterns and develop mathematical models that capture the underlying dynamics of complex systems.
For example, in finance, AI-powered mathematical models can analyze market data, identify trends, and make predictions about stock prices or market conditions. These models can help investors make informed decisions and manage risks more effectively. In engineering, AI-powered simulations can accurately model fluid dynamics, structural analysis, and electromagnetic interactions, allowing engineers to design and optimize complex systems with greater precision.
Furthermore, AI can also assist in solving partial differential equations (PDEs), which are fundamental equations in fields such as physics and engineering. PDEs describe the behavior of various physical phenomena, from heat transfer to wave propagation. Solving PDEs analytically can be challenging, if not impossible, in many cases. AI algorithms, such as neural networks and deep learning, can approximate the solutions to PDEs, providing accurate and efficient numerical solutions.
In conclusion, AI-powered mathematical modeling and simulations enable researchers to gain deeper insights into complex systems, make accurate predictions, and optimize designs. This not only enhances our understanding of the natural world but also drives innovation and progress in various scientific and engineering fields.
Enhancing mathematical education with AI
AI is not only transforming the practice of mathematics but also revolutionizing the way it is taught and learned. Traditional classroom settings often struggle to cater to the diverse learning needs and preferences of individual students. However, with the advent of AI, personalized and adaptive learning experiences are now within reach.
AI-powered learning platforms can assess students' strengths, weaknesses, and learning styles, allowing for tailored instruction and personalized feedback. These platforms can adapt to individual students' progress and provide targeted recommendations and resources to address specific areas of improvement. This individualized approach accelerates the learning process and enhances students' understanding and retention of mathematical concepts.
One notable application of AI in mathematical education is intelligent tutoring systems. These systems use AI algorithms to provide personalized guidance and support to students. By analyzing students' responses, the system can identify misconceptions, provide targeted explanations, and offer additional practice materials. This real-time feedback and guidance enable students to learn at their own pace and overcome challenges more effectively.
Furthermore, AI can also facilitate collaborative learning experiences. Through AI-powered platforms, students can engage in virtual discussions, solve problems together, and share their insights. This collaborative approach fosters a sense of community and enhances students' problem-solving and critical thinking skills.
Despite these advancements, it is important to note that AI should not replace human teachers. Rather, it should complement their expertise and provide additional support. Human teachers play a crucial role in understanding students' individual needs, fostering creativity, and inspiring a love for mathematics. AI can assist in automating administrative tasks, grading assignments, and providing personalized recommendations, allowing teachers to focus on more meaningful interactions with students.
In summary, AI-powered educational tools and platforms have the potential to revolutionize mathematical education by providing personalized instruction, adaptive learning experiences, and intelligent tutoring systems. These advancements not only enhance students' learning outcomes but also empower teachers to create engaging and effective learning environments.
AI algorithms for data analysis and pattern recognition in mathematics
In the age of big data, AI algorithms are playing a crucial role in analyzing and extracting insights from vast amounts of data. Mathematics, with its emphasis on patterns and relationships, is particularly suited to benefit from AI-powered data analysis and pattern recognition techniques.
AI algorithms, such as machine learning and data mining, can identify patterns and trends in large datasets, uncovering hidden relationships and providing valuable insights. In mathematics, these algorithms can be used to analyze mathematical structures, explore mathematical properties, and discover new theorems.
For example, in number theory, AI algorithms have been used to search for large prime numbers or find patterns in the distribution of primes. These discoveries contribute to our understanding of one of the oldest and most fundamental areas of mathematics. In graph theory, AI algorithms can analyze complex networks and identify key properties, such as centrality or connectivity. These insights have applications in various fields, including social network analysis and transportation planning.
Moreover, AI algorithms can also assist in solving mathematical puzzles and conjectures. In 2019, an AI algorithm developed by mathematicians at the University of Liverpool successfully solved the 40-year-old Erdős discrepancy problem, which involves finding patterns in the coloring of integers. This breakthrough demonstrates the potential of AI in solving complex mathematical problems and uncovering new mathematical knowledge.
In summary, AI algorithms for data analysis and pattern recognition are empowering mathematicians to explore mathematical structures, uncover hidden relationships, and make new discoveries. By leveraging the power of big data and AI, mathematics is entering a new era of exploration and innovation.
AI-driven mathematical research and discoveries
AI is not only assisting mathematicians in solving complex problems but also actively contributing to mathematical research and discoveries. By leveraging AI algorithms and tools, mathematicians can explore uncharted territories, make conjectures, and validate hypotheses.
One area where AI has shown remarkable promise is in the field of combinatorics. Combinatorics deals with counting, arranging, and analyzing discrete objects and structures. AI algorithms, such as reinforcement learning and genetic programming, can generate new combinatorial objects and explore their properties. These generated objects can lead to the discovery of new combinatorial structures or provide insights into existing ones.
Furthermore, AI algorithms can also assist in proving mathematical theorems or conjectures. In 2016, an AI algorithm developed by researchers at the University of Texas at Austin proved the Robbins conjecture, a long-standing problem in graph theory. The algorithm generated a proof that was subsequently verified by human mathematicians. This collaboration between AI and human mathematicians showcases the potential of AI in assisting and accelerating mathematical research.
Moreover, AI can also facilitate interdisciplinary collaborations and promote cross-pollination of ideas. By analyzing data from different fields, AI algorithms can identify connections, analogies, and similarities between seemingly unrelated mathematical concepts. This interdisciplinary approach can lead to new insights, novel approaches, and unexpected breakthroughs.
In conclusion, AI-driven mathematical research and discoveries are reshaping the landscape of mathematics, allowing mathematicians to explore new frontiers, validate conjectures, and collaborate across disciplines. The synergy between AI and mathematics holds great promise for unraveling the mysteries of the mathematical universe.
AI-powered tools and software for mathematicians
AI-powered tools and software are transforming the way mathematicians work, enabling them to tackle complex problems, explore mathematical structures, and visualize abstract concepts.
One area where AI has made significant strides is in mathematical proof verification. Proving mathematical theorems is a complex and time-consuming process that often involves intricate logical reasoning. AI algorithms, such as automated theorem provers and proof assistants, can assist mathematicians in verifying the correctness of proofs or even generate new proofs.
Moreover, AI-powered visualization tools are helping mathematicians gain deeper insights into abstract concepts and complex mathematical structures. These tools can transform complex mathematical equations into interactive visual representations, allowing mathematicians to explore patterns, make conjectures, and test hypotheses. By visualizing mathematical ideas, mathematicians can communicate their findings more effectively and inspire new lines of research.
Furthermore, AI algorithms can also assist mathematicians in generating new conjectures or hypotheses. By analyzing patterns, structures, and relationships in mathematical data, AI algorithms can suggest potential conjectures or provide insights into unsolved problems. These AI-generated conjectures can serve as starting points for further investigation and exploration.
In addition, AI-powered software can automate repetitive mathematical computations, allowing mathematicians to focus on higher-level thinking and problem-solving. These software tools can perform calculations, solve equations, and manipulate mathematical objects with speed and accuracy. By automating routine tasks, mathematicians can devote more time and energy to creative and innovative endeavors.
In summary, AI-powered tools and software are empowering mathematicians by automating routine tasks, assisting in proof verification, visualizing abstract concepts, and generating new conjectures. These tools not only enhance productivity but also stimulate creativity and innovation in the field of mathematics.
Ethical considerations in AI and mathematics
While AI holds great promise in revolutionizing mathematics, it is important to address the ethical considerations associated with its use in this domain.
One of the ethical concerns is the potential bias in AI algorithms. AI algorithms are trained on large datasets, which may contain biased or incomplete information. If these biases are not properly addressed, AI algorithms can perpetuate and amplify existing biases in mathematical research and education. It is crucial to ensure that AI algorithms are trained on diverse and representative datasets and that their outputs are thoroughly scrutinized for any biases.
Another ethical consideration is the impact of AI on the job market for mathematicians. As AI algorithms become more capable of solving complex mathematical problems, there is a concern that traditional mathematical roles may be automated, leading to job displacement. It is important to strike a balance between leveraging AI to enhance mathematical research and education while also preserving the role of human mathematicians.
Additionally, the ethical use of AI in mathematics requires transparency and accountability. AI algorithms should be open to scrutiny, and their decision-making processes should be explainable. Mathematical research and discoveries facilitated by AI should be clearly documented and validated by human mathematicians. This ensures the integrity of mathematical knowledge and prevents the propagation of false or misleading results.
Moreover, the ethical considerations extend to the ownership and privacy of mathematical data. As AI algorithms rely on large datasets, it is vital to protect the privacy and confidentiality of sensitive mathematical data. Proper data governance and privacy regulations should be in place to ensure that mathematical data is used responsibly and ethically.
In conclusion, the ethical considerations surrounding AI in mathematics encompass addressing biases in algorithms, preserving the role of human mathematicians, ensuring transparency and accountability, and safeguarding the privacy of mathematical data. By addressing these ethical concerns, we can harness the full potential of AI in advancing mathematical research and education while ensuring fairness and integrity.
Challenges and limitations of AI in mathematics
While AI has tremendous potential in revolutionizing mathematics, there are also challenges and limitations that need to be addressed for its effective integration in this domain.
One of the challenges is the explainability and interpretability of AI algorithms. AI algorithms, particularly those based on deep learning, can be highly complex and opaque. Understanding how these algorithms arrive at their solutions or predictions can be challenging, making it difficult to validate their results or trust their outputs. Researchers are actively working on developing explainable AI algorithms that provide transparent decision-making processes and can be understood by human mathematicians.
Another challenge is the need for large amounts of quality data. AI algorithms rely on extensive datasets for training and learning. However, in some areas of mathematics, obtaining such datasets may be challenging or even impossible. For example, in pure mathematics, where proofs and theorems are based on logical reasoning rather than empirical data, the availability of large datasets is limited. Developing AI algorithms that can work effectively with limited data or that can generate synthetic data is an ongoing research area.
Furthermore, the limitations of computational power and resources pose challenges in applying AI to certain mathematical problems. Some mathematical problems, such as the Riemann Hypothesis or the Navier-Stokes equations, are notoriously difficult and require massive computational resources to solve. While AI can provide valuable insights and assist in these problems, it may not be able to provide definitive solutions due to the limitations of computational resources.
Moreover, the lack of domain expertise in AI algorithms can lead to erroneous or misleading results. AI algorithms are only as good as the data they are trained on and the assumptions they make. Without a deep understanding of the mathematical domain, AI algorithms may generate incorrect or nonsensical results. It is important to combine the expertise of mathematicians with the power of AI to ensure accurate and meaningful outcomes.
In summary, challenges such as explainability, data availability, computational limitations, and domain expertise need to be addressed to fully leverage the potential of AI in mathematics. By overcoming these challenges, we can harness the power of AI to tackle complex mathematical problems, make new discoveries, and advance the field of mathematics.